In recent years, Deep Learning (DL), aiming at learning multiple-level of representations directly from data, has emerged as a powerful tool for different computer vision tasks. In the field of medical imaging, DL is becoming the state-of-the-art, with a huge potential in several medical applications such as image segmentation, image registration, early anomalies detection, etc.
Deep Learning for Radiation Therapy
Check the External Radiation Therapy section.
Automatic pectoralis and subcutaneous fat segmentation
Body composition measurements are considered very valuable for patient treatment as it has been recognized that the way fat and muscle are distributed in the body has a critical repercussion in several numbers of conditions. Body mass index (BMI) or fat-free mass (FFM) are examples of indicators of relevant clinical outcomes. Additionally, it has been observed that computer tomography (CT) measures of pectoralis muscle area (PMA) and subcutaneous adipose tissue (SAT) on a single slice are significantly related to COPD outcomes and could be more clinically relevant than BMI or FFM. Up to date, these body composition values have been measured through manual segmentation.
We propose a complete automated segmentation workflow to obtain clinically relevant PMA and SAT measurements from CT images. Therefore, we propose a fully automated method using CNNs which is divided into two steps. First, a structure detection method which uses CNNs to automatically detect a reproducible axial slice from a CT where pectorals and fat are visible, followed by an established selection criteria. Second, the slice selected is used to predict body composition measurement through the segmentation model based on FCNNs.
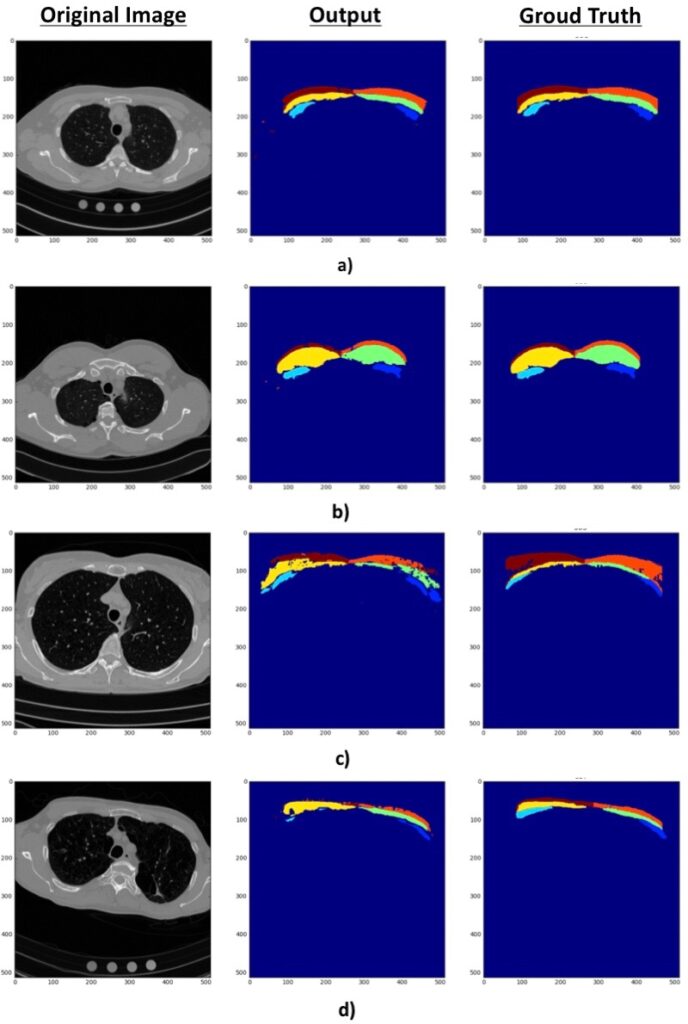
- R. Moreta-Martinez, J. Onieva-Onieva, J. Pascau, R. San Jose Estepar. Pectoralis muscle and subcutaneous adipose tissue segmentation on CT images based on convolutional networks. In: CARS 2017 – Computer Assisted Radiology and Surgery Proceedings of the 31th International Congress and Exhibition, Barcelona, Spain, June 20–24, 2017. Int J CARS 12, 31–32 (2017) [doi]
Automatic segmentation of organs at risk for prostate cancer radiotherapy
In prostate cancer, radiotherapy (RT) involves irradiation of the tumor but also of neighboring organs at risk, inducing toxicity and, consequently, impacting patients’ quality of life. Therefore, the next frontier of this field is to devise personalized treatment plans aimed at reducing RT-induced toxicities.
Our group is currently working on the development of segmentation algorithms for organs at risk and sub-organ regions identified as predictive for toxicity, under the project Personalized Planning in Radio Therapy Through Integrative Modeling of Local Dose Effect and New Dosimetric Constraints.
Related publications
- R. Moreta-Martinez, J. Onieva-Onieva, J. Pascau, R. San Jose Estepar. Pectoralis muscle and subcutaneous adipose tissue segmentation on CT images based on convolutional networks. In: CARS 2017 – Computer Assisted Radiology and Surgery Proceedings of the 31th International Congress and Exhibition, Barcelona, Spain, June 20–24, 2017. Int J CARS 12, 31–32 (2017) [doi]